In the past few months, we’ve seen growing excitement around AI and its potential to reinvent the way we work. However, many unanswered questions remain about practical business applications of the technology, particularly for heavily regulated industries like finance. Having spent the last decade improving finance firms’ efficiency through technology, we wanted to dig deeper into how AI could impact the industry.
We spoke with Julien Villemonteix, our CEO and former Chief Product Officer who wrote the source code for UpSlide over 13 years ago, to gather his insights this revolutionary technology. We wanted to find out:
- What are the main use cases for AI in finance?
- What are the key risks to consider?
- Is AI a passing craze, or will it change how we work forever?
Read on to discover everything you need to know about AI, Microsoft 365 Copilot, and what this means for the finance industry.
TL;DR
The main use cases for AI in finance include: translating legacy code, accelerating customer query resolution, and streamlining document generation. AI can automate low-value tasks, freeing professionals for more valuable work.
AI might indirectly help businesses achieve their strategic goals; it reduces document creation time, leading to higher employee output. It also allows for enhanced client relationships, data insights, and strategic decision-making.
However, AI has limitations, such as the need for extensive data, potential inaccuracies, security risks, and compliance concerns. Microsoft Copilot aims to address some of these issues.
What are the different applications of AI in the finance industry?
Julien Villemonteix: AI is a “suitcase word” – one which you can pack multiple meanings inside – so let’s start by defining what we mean when talking about AI.
We’re focusing on text generation and Large Language Models (LLMs), which have been at the center of attention since OpenAI launched its popular ChatGPT in late 2022.
Everyone is trying to ascertain what the future of AI could look like; however, some clear use cases for AI in finance are starting to emerge:
- For R&D and software engineering teams: translating legacy code into multiple languages
- For internal support teams: accelerating the resolution rate of internal queries through Interactive Voice Response (IVR)
- For front-office and marketing teams: improving the efficiency of document generation
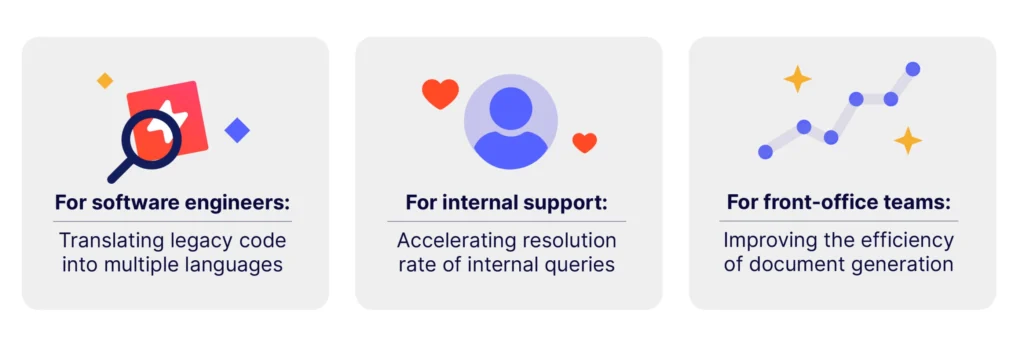
Generative AI could deliver significant value when deployed in certain use cases, particularly on employees’ productivity. If integrated effectively, it could automate some of the lower-value-added tasks, and free finance professionals to work on the more valuable parts of their roles.
However, we can’t naively believe that AI will solve all business inefficiencies, nor will it have a significant impact right away.
So if you’re looking for a more tangible solution to your pain points, we suggest exploring other ways to achieve this (e.g. if it’s inefficient workflows in Microsoft Office – perhaps integrate an automation software to streamline document creation as a starting point).
How will AI help with wider strategic business goals?
JV: Finance professionals currently spend too much time and resource creating documents in the Microsoft Office suite.
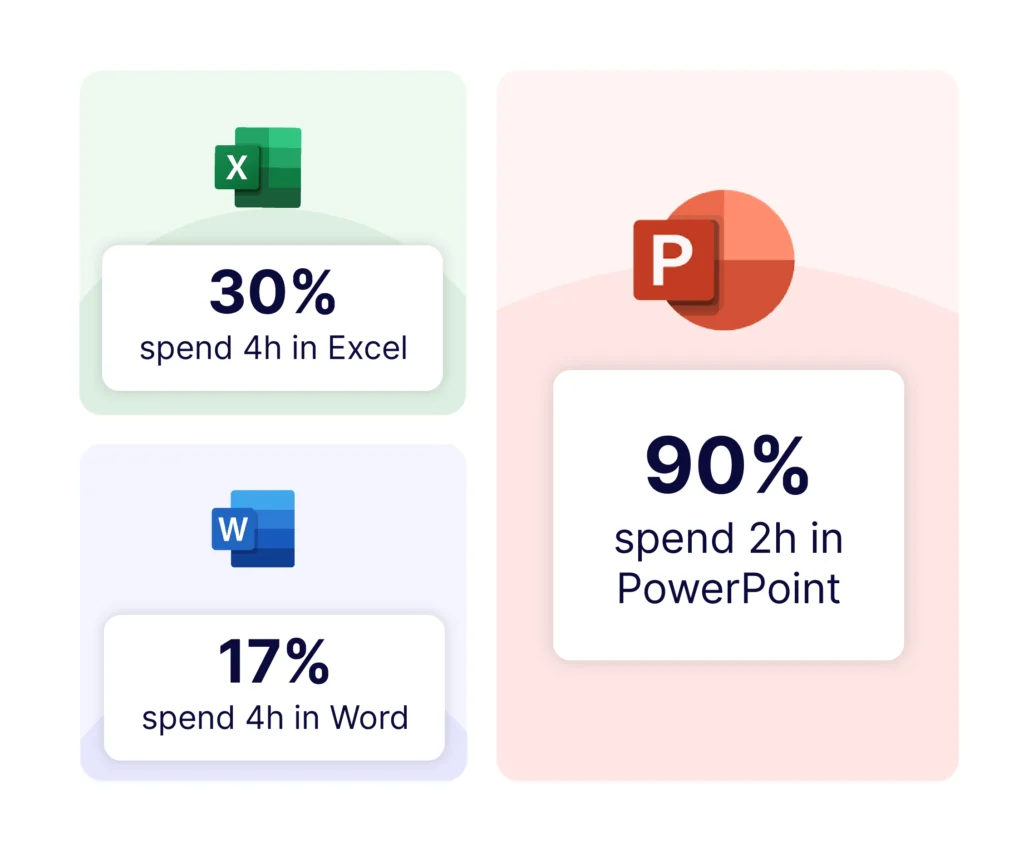
As mentioned, AI could reduce the time spent creating documents by generating content and summarizing data faster, resulting in higher employee output.
But the real cherry on top is what your workforce could do with the time gained; there might be more opportunities to enhance client and customer relationships, maximize data insights and time to spend on strategic decision-making – all of which positively impacts the bottom line.
This more satisfying, purpose-led workload will undoubtedly lead to a happier, more fulfilled workforce, improving employee retention rates.
Is AI a passing craze, or will it change the way we work forever?
JV: Aside from the productivity benefits, AI will very likely have a huge impact on the future of user interface development and provide new possibilities for users to interact with software. For example, instead of clicking multiple times to create a two-column slide and insert a blank chart in the right column, they can ask AI to do it.
Plus, with AI, software can better understand the user context and leverage it in the interface. For example, a particular software might’ve had dozens of buttons permanently visible. Now with AI, it could analyze what the user is doing within the application and only show the relevant buttons when they need them.
As the CEO of a software company, I’m particularly excited to see what the future of user interface could look like with AI.
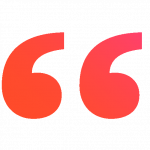
With AI, software can better understand the user context and leverage that in the user interface.
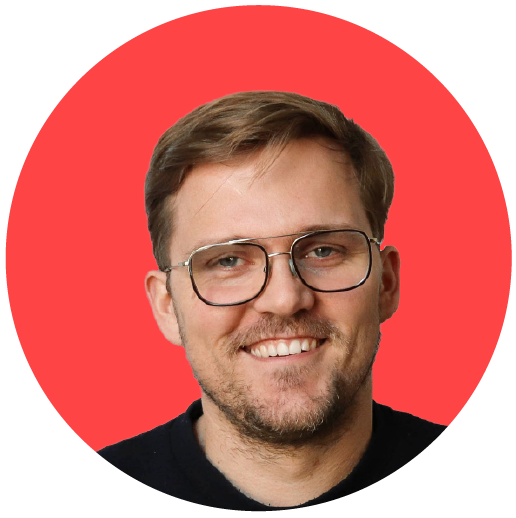
Julien Villemonteix
CEO
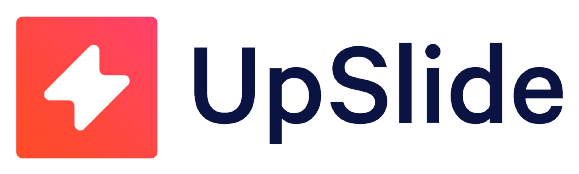
What are the limitations of AI?
JV: AI has a lot of potential, but like any technology, it has limitations.
The first question is: how much data must we feed into the models before we can rely on it to concretely help with decision-making? AI needs a lot more input and experience than a human to deliver the same results, so when you have to make a decision with limited information, for now, it’s better to rely on human input over LLMs.
The second limitation is that LLMs will continue to hallucinate information (generate factually incorrect text) for the foreseeable future, and we can’t guarantee the results will be accurate or true. Until the models have matured, human input will still be required to spot these hallucinations and avoid presenting inaccurate data. This is summarized with Microsoft’s aptly named Copilot – it’s a co-pilot, not an autopilot.
Plus, there are also concerns around security and compliance risks. For example:
- AI is not yet sophisticated enough to understand the complex nuances of software development, making its code vulnerable and more susceptible to malware.
- Chatbots like ChatGPT collect and store user data such as IP address, browser information and activities, which might be shared with third-party entities.
- It requires employees to share information (via prompts) to generate tailored results. However, employees might unknowingly share confidential corporate or client information, which could have significant consequences if leaked in a data breach.
A number of big banks like JP Morgan and Goldman Sachs have prohibited or limited employee use of ChatGPT to mitigate these risks.
However, after hearing the recent announcements at Microsoft Build, we believe that Microsoft’s Copilot is well-positioned to overcome some of the concerns mentioned.
Copilot’s security measures
JV: Unlike ChatGPT and many other generative AI, Copilot doesn’t rely on your users’ data to train the LLM beyond organizational use, meaning no sensitive data will sit outside of resources you control.
Plus, it’s built upon the software and applications that financial professionals use daily, which have already been approved by a firm’s IT team.
In a nutshell, if your business trusts SharePoint and Azure already, you should be able to trust Copilot.
Can Copilot address the needs of finance professionals in Office 365?
JV: We anticipate that Copilot could greatly impact the daily workflows of the average Microsoft Office user, helping generate emails and summarize meetings and documents.
However, we’re doubtful it will completely reinvent how financial professionals work. These individuals must create high-quality, accurate and compliant documents, and despite Copilot’s advanced capabilities, the complexity of tasks required could be too big of an obstacle.
Additionally, even if Copilot helps with some everyday inefficiencies, it won’t help to tackle some of the broader challenges when creating pitchbooks, such as guaranteeing brand consistency.
How does UpSlide sit alongside Microsoft Copilot in the Office 365 ecosystem?
JV: As a long-standing Microsoft Solutions Partner, we’re excited to see them position themselves at the centre of AI conversations.
In time, Microsoft 365 will be able to offer an AI solution that will satisfy the needs of the average Office worker and streamline inefficient workflows. However, our mission at UpSlide is to keep providing value for employees in financial and professional services alongside Copilot.
Copilot – and AI – won’t replace our finance-dedicated features and integrations like the Excel and PBI to PowerPoint Links or our in-app Tombstone Library. It may make document generation easier, but not with the level of reliability and support we can guarantee.
We’re excited to work alongside Copilot and pledge to continue understanding our finance users’ workflows, identify pain points, and answer their specific needs alongside AI.
Discover how UpSlide will work with Copilot
Read our recent blog to understand UpSlide’s positioning on AI and how we’ll continue to deliver value to the finance industry alongside Microsoft Copilot.
What’s next for AI?
There will undoubtedly be more exciting developments for AI in the next few months: Microsoft might announce the general release date for Copilot, many more banking titans (JP Morgan Chase & Co) could take the plunge and develop AI-powered technology in-house, or we might get more of an idea of how this will impact everyday life.
There’s lots of promise on the horizon, and we’re eager to see what’s in store for AI, particularly Microsoft Copilot.
In the meantime, follow us on LinkedIn for more regular updates on AI in the finance industry and automating repetitive tasks in Microsoft 365.